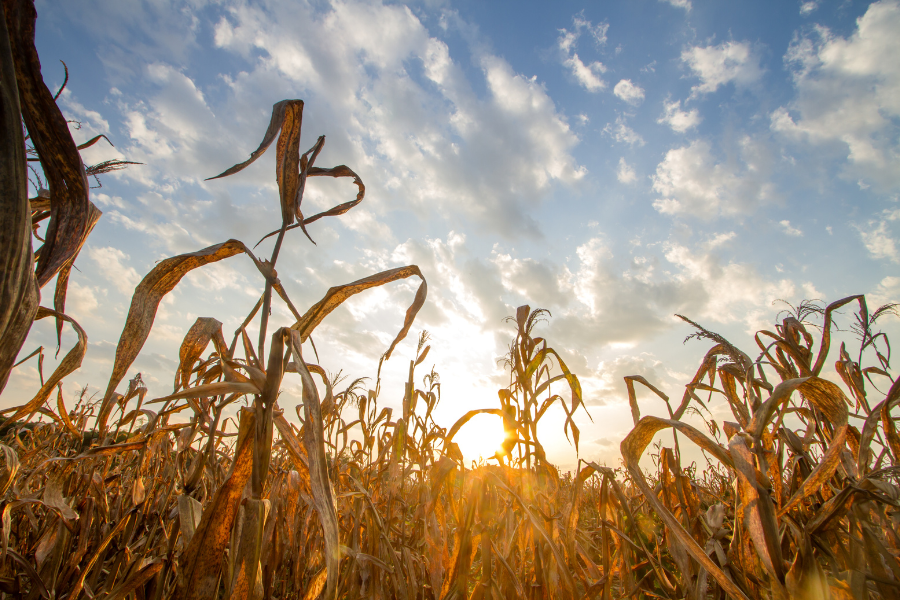
The shifting dynamics of climate are reshaping global agriculture, creating unprecedented challenges for crop production. For stakeholders, from policymakers to precision agriculture innovators, mitigating these impacts is critical. While traditional practices rely heavily on experience and historical knowledge, the increasing unpredictability of climate patterns demands a new paradigm.
This blog delves deep into how advanced data analytics and geospatial intelligence enable actionable insights to tackle the intricate relationship between climate variability and crop yield.
The Complex Dynamics of Climate Variability and Crop Yield
Climate variability encompasses more than just extreme weather; it involves shifts in temperature ranges, seasonal precipitation patterns, and frequency of anomalies like El Niño or La Niña events. These shifts significantly influence crop growth cycles, soil health, pest dynamics, and even pollination patterns.
Critical Variables at Play:
- Temperature Anomalies: Even slight temperature deviations can reduce crop yields. For instance, wheat and corn yield drops by 6% for every degree of warming during the growing season.
- Erratic Rainfall: Over 60% of agricultural land worldwide is rainfed, making irregular rainfall patterns a significant disruptor.
- Soil Health Erosion: Changes in climate directly impact organic matter decomposition rates, which alters soil fertility.
- Increased Pest Pressure: Warmer climates expand the range and lifespan of pests, leading to higher crop losses.
Understanding how these interconnected variables affect specific crops requires granular data and the ability to process it effectively.
Advanced Analytics for Precision Agriculture
- Geospatial Data Integration
The advent of geospatial intelligence has revolutionized agricultural planning. By layering satellite imagery with climate data, farmers can understand localized impacts of climate variability. For example, NDVI (Normalized Difference Vegetation Index) maps combined with temperature and rainfall trends provide a real-time assessment of crop health at the micro-level.
Use Case:
In Australia, where wheat is a major crop, integrating remote sensing data with seasonal climate forecasts has enabled farmers to adjust planting dates, reducing the risk of crop failure by 18% in areas prone to late frosts.
- Predictive Modeling for Climate Resilience
Predictive models use machine learning to process vast datasets, including historical weather patterns, soil properties, and genetic crop data. These models can simulate multiple scenarios:
– Estimating the yield potential under varying climatic conditions.
– Identifying the most resilient crop varieties.
– Forecasting disease outbreaks linked to climatic changes.
Technical Insight:
For instance, regression models combined with neural networks can predict rice yield with a margin of error below 5%, even under variable monsoon conditions.
- Hyperlocal Weather Forecasting
Traditional weather forecasts often fail to capture the granularity needed for agricultural decision-making. Hyperlocal forecasting uses AI to downscale global weather models, offering insights at the farm level.
Impact:
– Optimized irrigation schedules reduce water waste.
– Timely pest control interventions based on humidity and temperature shifts.
– Enhanced post-harvest planning by predicting drying conditions.
Climate-Informed Crop Diversification
Relying on a narrow set of crops inherently increases vulnerability to climatic shocks. In regions where farmers primarily grow one or two crops, a sudden shift in climate conditions—whether it’s prolonged droughts, erratic rainfall, or unseasonably high temperatures—can lead to catastrophic yield losses. Such risks are not only damaging to the environment but also threaten food security and livelihoods. For this reason, crop diversification is a proven strategy to mitigate the impact of climate variability, and data-driven approaches are proving essential in guiding these diversification decisions.
The Need for Diversification in an Unpredictable Climate
Historically, crop diversification was a technique largely based on traditional knowledge and experience. However, in today’s rapidly changing climate, the unpredictability of seasonal patterns and the increasing frequency of extreme weather events demand a more scientific approach. Diversification becomes an insurance policy, reducing a farmer’s reliance on the success of a single crop and enhancing resilience against unexpected climatic shifts.
For instance, crops such as maize, wheat, and rice are highly sensitive to variations in temperature and precipitation. Under unpredictable climatic conditions, their yields may decline significantly. By diversifying crop choices to include drought-resistant varieties or those suited to different climatic zones, farmers can buffer against crop failure and optimize productivity.
Data-Driven Crop Diversification Strategies
To implement effective crop diversification, it’s essential to use precise, localized data to guide decisions. Climate forecasts, coupled with soil suitability maps, can provide farmers with insights into which crops are best suited to the evolving climate of their region. These tools allow for the identification of alternative crops that are better equipped to handle specific environmental conditions, thus improving both yield stability and sustainability.
Key Data Components Driving Diversification:
- Climate Forecasts and Projections: Detailed, localized climate forecasts provide predictive insights into future conditions, such as rainfall patterns, temperature fluctuations, and the likelihood of extreme weather events. This data helps farmers identify crops that will thrive under these conditions.
- Soil Suitability Mapping: Advanced soil mapping technologies use data on soil texture, pH, and nutrient levels to identify which crops can grow optimally in specific soil types. By considering both climate conditions and soil health, farmers can make more informed decisions about which crops will not only survive but thrive in the face of climate variability.
- Historical Yield Data: Integrating historical data on crop yields with climate and weather patterns allows farmers to see which crops have been most resilient in the past under varying climatic conditions. This helps in identifying crop varieties that have performed well during similar weather patterns, enabling better forecasting of future outcomes.
- Pest and Disease Risk Modeling: Shifts in temperature and precipitation can alter the prevalence and distribution of pests and diseases. Predictive models can identify potential risks and inform farmers of crop varieties with greater resistance to these threats, further enhancing diversification strategies.
Benefits of Climate-Informed Diversification
- Improved Resilience: Diversification reduces the risk that all crops will fail in response to a single climatic event. By planting a range of crops suited to various climatic conditions, farmers create a buffer that increases their chances of success regardless of short-term weather changes.
- Higher Overall Yield Stability: While individual crops may suffer due to adverse weather, the combined yield from a diversified set of crops is likely to be more stable. Some crops might thrive during dry spells, while others flourish with excess rain, ensuring a more consistent and reliable harvest.
- Risk Mitigation: With an increase in extreme weather events such as floods, droughts, and heatwaves, climate-informed diversification can help buffer against these risks. By planning for variability rather than relying on a fixed set of crops, farmers ensure greater long-term viability of their operations.
- Economic Benefits: Climate-informed crop diversification can open new market opportunities by expanding the types of crops a farm can produce. Additionally, as demand for certain crops may fluctuate due to changing climates, diversifying production allows farmers to tap into emerging markets, thus improving income stability.
Challenges in Scaling Data-Driven Agriculture
Despite its promise, adopting a data-driven approach is not without hurdles:
- Fragmented Data Ecosystems: Data often resides in silos across weather stations, private companies, and research institutions, limiting its integration.
- High Entry Costs: Many small-scale farmers cannot afford advanced sensing equipment or premium analytics services.
- Lack of Technical Know-How: Transforming raw data into actionable insights requires expertise that is still scarce in many regions.
- Climate Data Gaps: In many parts of the Global South, there is insufficient historical climate data to train robust predictive models.
To address these challenges, partnerships across sectors—government, academia, and private industry—are essential.
Conclusion
The relationship between climate variability and crop yield is intricate and multifaceted. However, with the right tools and strategies, the agriculture sector can turn challenges into opportunities. By leveraging advanced data analytics, predictive models, and geospatial intelligence, we can not only mitigate the impacts of climate variability but also build a resilient food production system.
Envision Beyond is committed to driving this transformation. Whether you’re a farmer seeking to optimize your yield or a policymaker looking for scalable solutions, we provide the expertise and tools to guide you toward a sustainable agricultural future.